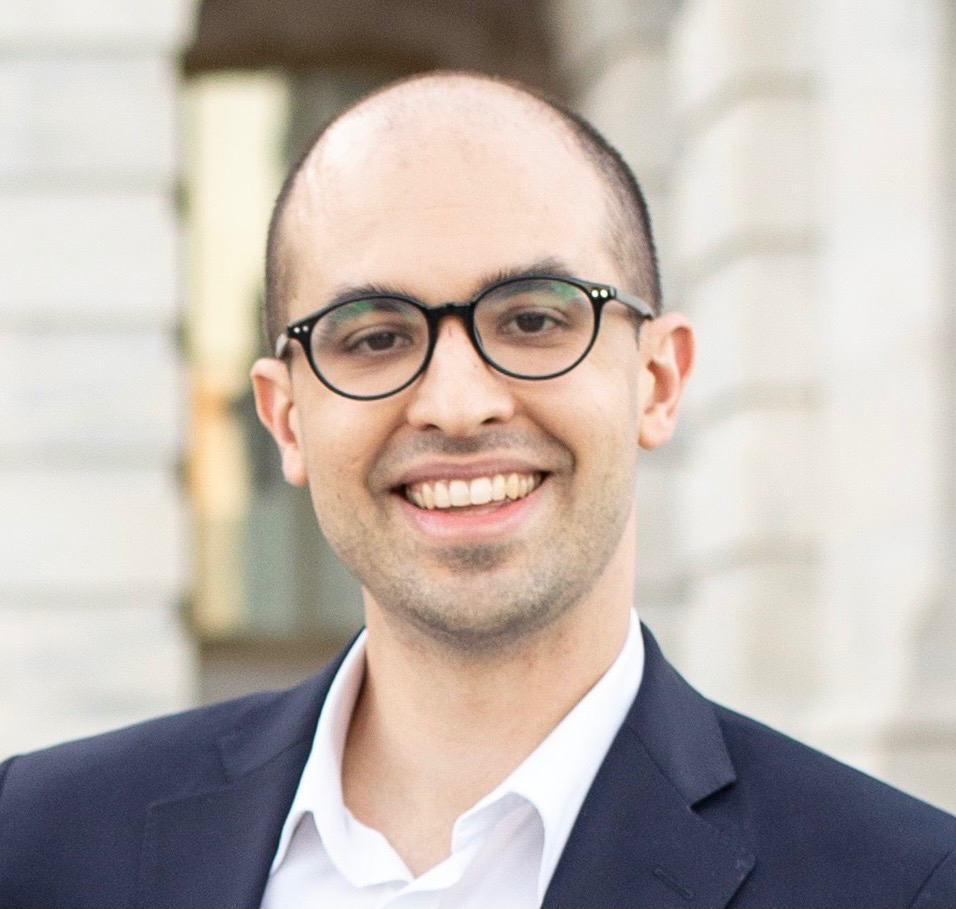
Matthew Marge
DEVCOM Army Research Laboratory
Time: 10/17/2022, 10:00am EST - 11:00am EST
Place: Hybrid
Topic: Robots in Situated Dialogue
Title: Robot Concept Learning in Situated Dialogue
Abstract: Intelligent agents that refer to and make use of the physical world, like robots, will be more able to adapt to new situations if they can learn concepts in real time from humans. This process forms an interactive dialogue loop between robots asking questions to learn more about the physical world and humans using natural language to teach them. In this talk, I will present findings from the Human-Robot Dialogue Learning project that explored these topics. Key accomplishments include (1) an improved understanding of how humans teach robots compared to other humans, (2) a first-of-its-kind corpus of questions that robots can use to learn from human teachers, and (3) real-time algorithms that enable robots to generate questions that maximize learning in a cognitive robotic architecture. The end result is the novel capability for intelligent agents to use situated dialogue and one-shot learning to acquire more information about their surroundings with human teammates.
Bio: Matthew Marge is a Senior Computer Scientist at DEVCOM Army Research Laboratory (ARL). He received the Ph.D. and M.S. degrees in Language and Information Technologies from the School of Computer Science at Carnegie Mellon University, the M.S. degree in Artificial Intelligence from the University of Edinburgh, and the B.S. degrees in Computer Science and Applied Mathematics and Statistics from Stony Brook University. Dr. Marge's research focuses on how robots and other artificial agents can establish common ground with people through dialogue. His current interests lie at the intersection of computational linguistics, human-machine interaction, and integrative AI systems, specializing in conversational AI. Dr. Marge is a recipient of the 2018 Office of the Secretary of Defense's Laboratory University Collaboration Initiative award, supporting his research on dialogue with robots. In addition to his position at ARL, he is an Adjunct Professor in the Computer Science and Linguistics Departments at Georgetown University.
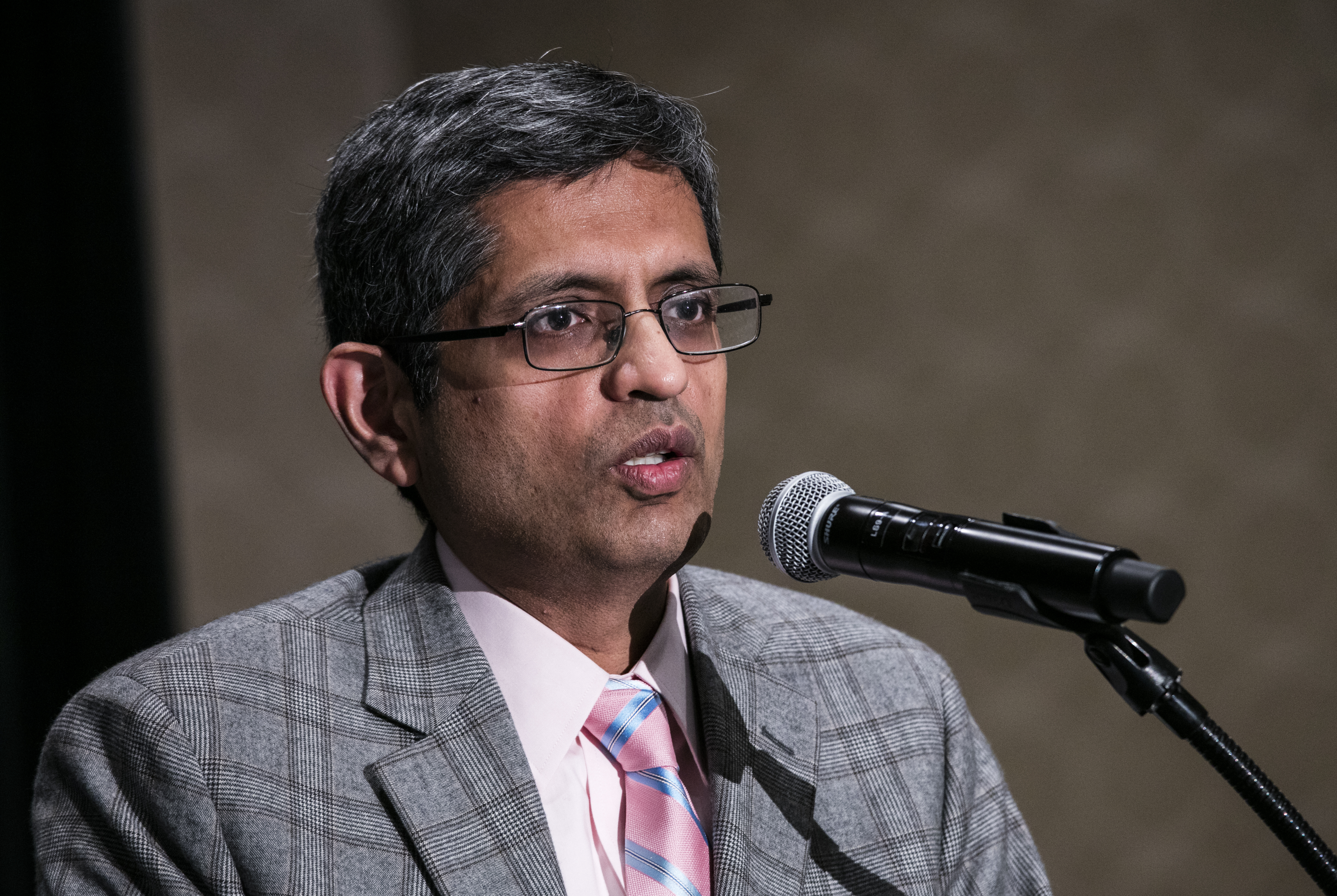
Ajay Divakaran
SRI International
Time: 10/12/2022, 3:00pm EST - 4:00pm EST
Place: Zoom
Topic: Multimodal NLP
Title: Using Hierarchies of Skills to Assess and Achieve Automatic Multimodal Comprehension
Abstract: Unlike current visual question answering (VQA), elementary school (K-5) teaching of reading comprehension has a graded approach based on a hierarchy of skills ranging from memorization to content creation. We take inspiration from such hierarchies to investigate both dataset creation and question answering techniques. First, we are currently creating a new visual question answering dataset that tests comprehension of VQA systems in a graded manner using hierarchical question answering with picture stories. Second, we investigate large language models such as GPT-Neo, the open version of GPT-3. We use Bloom’s Taxonomy of comprehension skills it to analyze and improve the comprehension skills of large pre-trained language models. Our experiments focus on zero-shot question answering, using the taxonomy to provide proximal context that helps the model answer questions by being relevant to those questions. We show that targeting context in this manner improves performance across 4 popular common sense question answer datasets. Third, we propose conceptual consistency to measure a LLM's understanding of relevant concepts. To compute it we extract background knowledge by traversing paths between concepts in a knowledge base and then try to predict the model's response to the anchor query from the background knowledge. We investigate the performance of current LLMs in a commonsense reasoning setting using the CSQA dataset and the ConceptNet knowledge base. While conceptual consistency, like other metrics, does increase with the scale of the LLM used, we find that popular models do not necessarily have high conceptual consistency. Finally, we present work on detection and removal of bias in common multimodal machine comprehension datasets. We hypothesize that this naturally occurring bias present in the dataset affects even the best performing model. We verify our proposed hypothesis and propose an algorithm capable of modifying the given dataset to remove the bias elements.
Bio: Ajay Divakaran, Ph.D., is the Technical Director of the Vision and Learning Lab at the Center for Vision Technologies, SRI International, Princeton. Divakaran has been a principal investigator for several SRI research projects for DARPA, IARPA, ONR etc. His work includes multimodal analytics for social media, real-time human behavior assessment, event detection, and multi-camera tracking. He has developed several innovative technologies for government and commercial multimodal systems. He worked at Mitsubishi Electric Research Labs during 1998-2008 where he was the lead inventor of the world's first sports highlights playback-enabled DVR, and several machine learning applications. Divakaran was named a Fellow of the IEEE in 2011 for his contributions to multimedia content analysis. He has authored two books, 140+ publications and 60+ issued patents. He received his Ph.D. degree in electrical engineering from Rensselaer Polytechnic Institute.
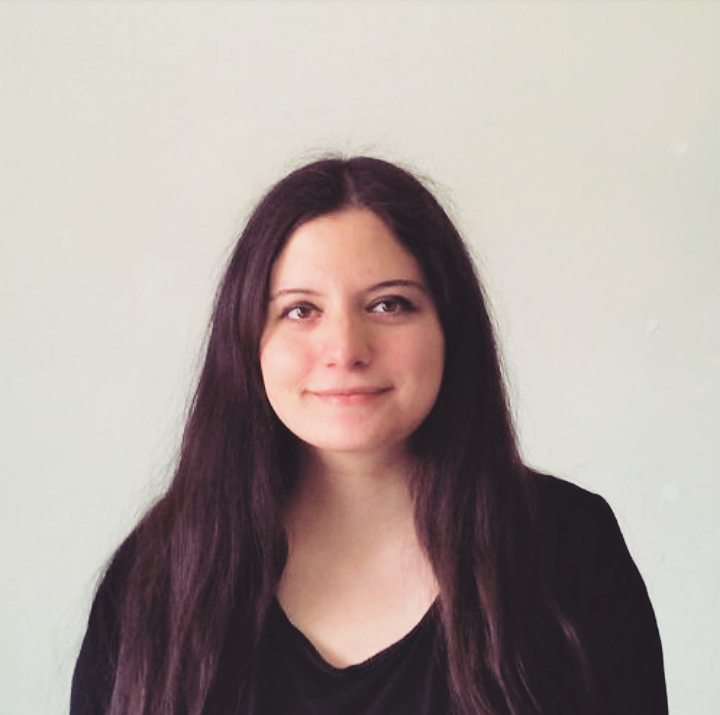
Ece Takmaz
University of Amsterdam
Time: 9/28/2022, 1:00pm EST - 2pm EST
Place: Zoom
Topic: Multimodal NLP
Title: Integrating Language, Vision and Human Gaze in Deep Neural Networks
Abstract: When speakers describe an image, there are complex visual and linguistic processes at work. For instance, speakers tend to look at an object before mentioning them. Inspired by these processes, we have developed the first models of image description generation informed by the cross-modal alignment between language and human gaze. We build our models on a state-of-the-art image captioning model, which itself was inspired by the visual processes in humans. Our results show that aligning gaze with language production would help generate more diverse and more natural descriptions that are better aligned with human descriptions sequentially and semantically. At the end of my talk, I would like to briefly touch upon another line of research where we quantify and implement possible strategies used by humans in generating and resolving incremental referring utterances in visual and conversational contexts.
Bio: Ece Takmaz is a 4th-year PhD candidate at the Institute for Logic, Language & Computation (ILLC), University of Amsterdam. She is part of the Dialogue Modelling Group led by Raquel Fernández. Ece received her B.S. degree in Computer Engineering from Bilkent University in 2012. She then obtained her M.S. degree in Cognitive Science from the Middle East Technical University in 2015. Afterwards, she received her M.S. degree in Artificial Intelligence from the University of Amsterdam in 2019. Her interests lie in Natural Language Processing and the integration of vision and language in tasks such as image captioning, visual question answering, and multimodal dialogue modelling. In addition, she works on incorporating cognitive signals such as eye-tracking data into multimodal models, inspired by the relation between visual and linguistic processes in human cognition.
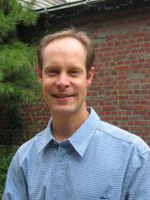
Mike White
The Ohio State University
Time: 4/1/2022, 2:00pm EST
Place: Zoom
Topic: Discourse and Generation
Title: The Case for Reviving Discourse Relations in NLG
Abstract: Neural methods for natural language generation arrived with much fanfare a few years ago with their promise of flexible, end-to-end trainable models. However, recent studies have revealed their inability to produce satisfactory output for longer or more complex texts. To address this issue, I will first discuss using tree-structured semantic representations that include discourse relations, like those used in traditional rule-based NLG systems, for better discourse-level structuring and sentence-level planning. I will then introduce a constrained decoding approach for sequence-to-sequence models that leverages this representation to improve semantic correctness on a conversational weather dataset as well as the E2E Challenge dataset. Next, I will examine whether it is beneficial to include discourse relations in the input to a neural reimplementation of a classic NLG system, Methodius, using both LSTM and pre-trained language models. Here, we find that these models struggle to correctly express Methodius’s similarity and contrast comparisons unless the corresponding RST relations are included in the inputs. Finally, to investigate whether discourse relations pay off in a broad coverage setting, I will report on experiments using pre-trained models with the Penn Discourse Tree Bank (PDTB) to generate texts with correctly realized discourse relations. Our results suggest that including discourse relation information in the input of the model significantly improves the consistency with which it produces a correctly realized discourse relation in the output, and also better matches the distribution of connective choices in the corpus.
Bio: Dr. Michael White is a Professor in the Department of Linguistics at The Ohio State University. His research has focused on NLG in dialogue with an emphasis on surface realization, extending also to paraphrasing for ambiguity avoidance and data augmentation in the context of Ohio State's virtual patient dialogue system. He co-organized the NSF Workshop on Shared Tasks in NLG which provided a crucial impetus for the initial shared tasks in NLG, and he was a co-organizer of the first surface realization shared task. Since 2018, Dr. White has been a frequent collaborator with conversational AI researchers at Facebook/Meta.
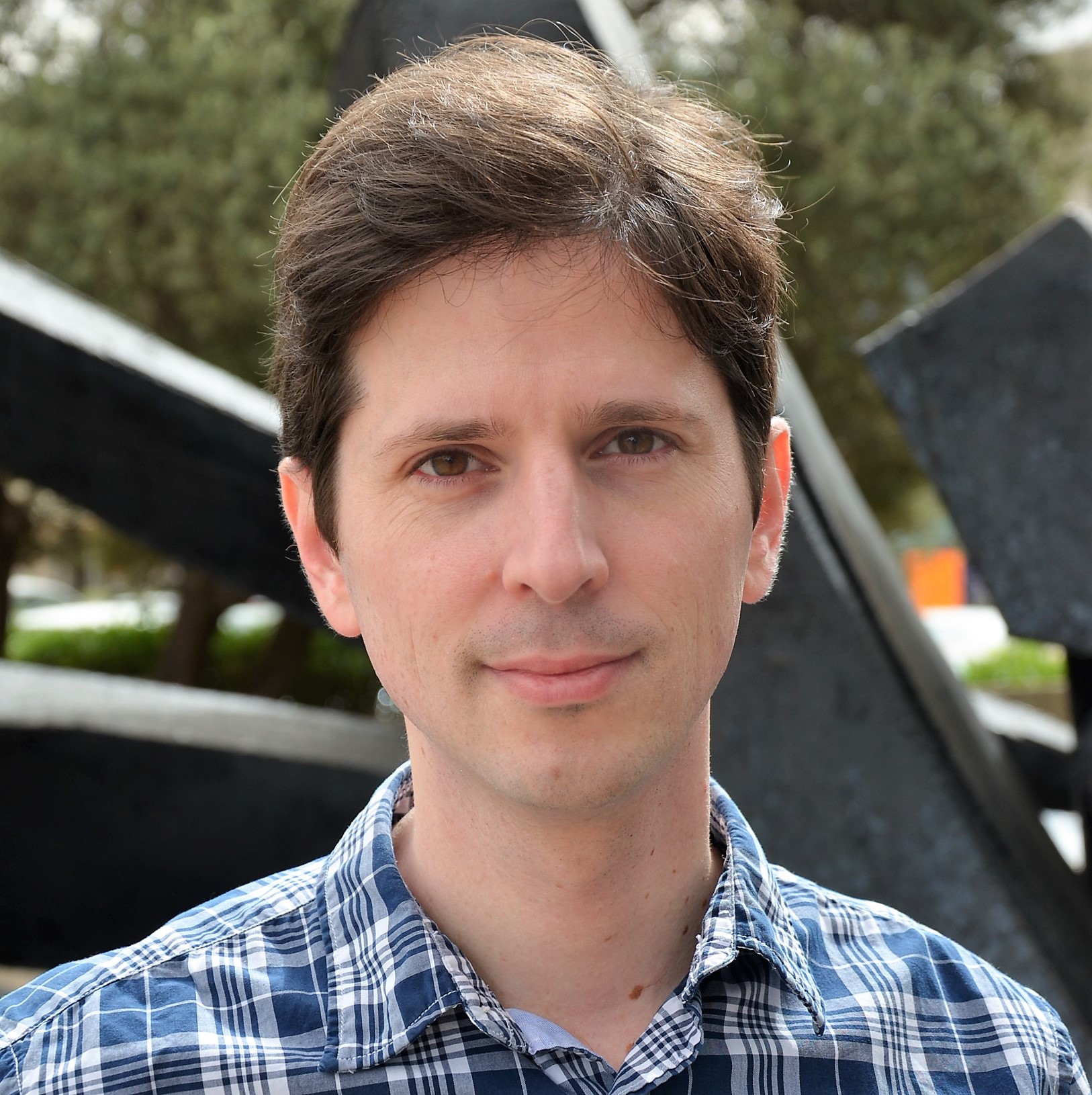
Amir Zeldes
Georgetown University
Time: 3/4/2021, 12:00pm EST
Place: Zoom
Topic: Computational Discourse Analysis
Title: Multilayer and Multi-Genre Models of Discourse
Abstract: Transformer-based word embeddings have led to impressive gains in traditional sequence labeling and classification tasks such as POS tagging, dependency parsing and NER. At the same time, models for discourse level understanding and generation beyond single sentences have been lagging substantially behind – not only in within-domain performance, but also in our understanding of what structured representations we want, and especially in our (in)ability to ensure that they will generalize to unseen data. In this talk I will give an overview of common building blocks for computational discourse models, focusing on entity mention models using anaphora resolution, and discourse relation graphs, such as RST trees. I will present data being created at Georgetown to facilitate truly domain general, reliable discourse modeling tools, ranging from the small but very richly annotated, multi-genre GUM corpus, to large-scale resources such as the AMALGUM corpus, built using multilayer-aware ensembles and active learning. Using a number of case studies, I will argue that domain-general discourse processing is needed where ad hoc ‘end to end’ neural approaches reach their limits, and that a multilayer, multi-genre approach is necessary to achieve this goal.
Bio: Amir Zeldes is Associate Professor of Computational Linguistics at Georgetown University, where he runs the Corpling Lab (Corpling@GU). His research focuses on discourse level phenomena beyond the sentence level, such as different types of anaphora and discourse relations, as well as low-resource NLP. He is active in building annotation interfaces, NLP tools, formats and standards for multilingual data, including work on projects such as Universal Dependencies, Universal Anaphora, and the DISRPT shared tasks (Discourse Relation Parsing and Treebanking). He is currently president of the ACL Special Interest Group on Annotation (SIGANN).
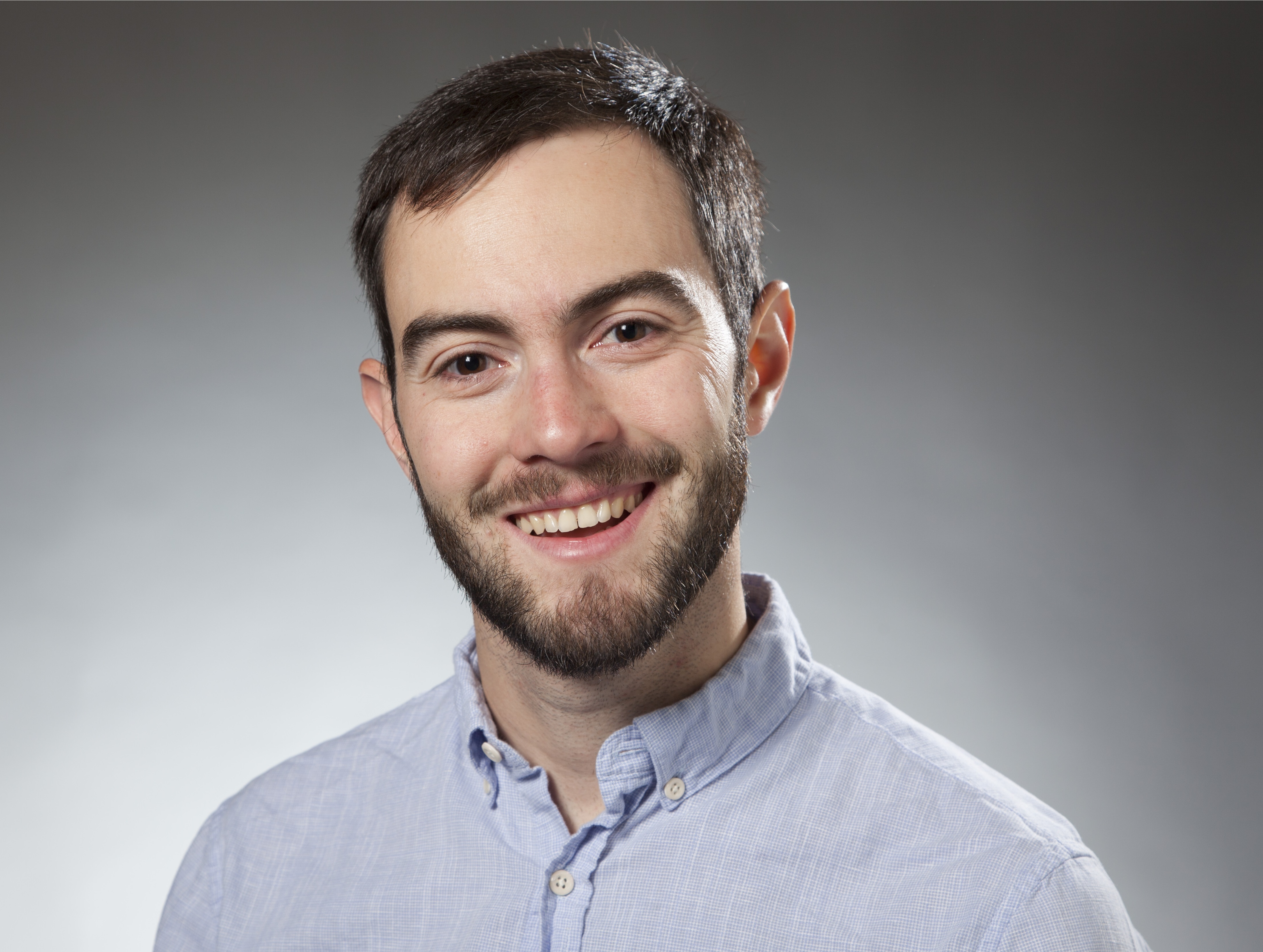
Dan Villarreal
University of Pittsburgh
Time: 2/4/2021, 12:00pm EST
Place: Zoom
Topic: Fairness of AI in Sociolinguistics
Title: Overlearning speaker gender in sociolinguistic auto-coding: metrics and remedies
Abstract: Sociolinguistic auto-coding is a method in which machine learning classifiers assign variants to variable data, such as classifying English non-prevocalic /r/ tokens as Present or Absent (e.g., in New York City English, car can be pronounced as "car" or "cah") based on acoustic features (Kendall et al. 2021; McLarty et al. 2019; Villarreal et al. 2020). While auto-coding promises opportunities for greater efficiency in the sociolinguistic research workflow, like other computational methods there are inherent concerns about this method’s fairness. Concerns about AI fairness have been raised, for example, in the American criminal justice system, where algorithms assessing the risk of a pretrial defendant may inadvertently use defendants’ race as a decision criterion (Angwin et al. 2016). It is an empirical question whether sociolinguistic auto-coding is subject to a similar fairness problem: by overlearning group-level characteristics cued by features in the training set, an auto-coder may make predictions about variants based not on legitimate cues to variant identity, but inadvertently on group membership (e.g., Kendall et al. 2021: 14). Such overlearning would be problematic for sociolinguistic work given the central importance of correlating speaker groups to differences in variable usage. The present research thus investigates fairness in sociolinguistic auto-coding. First, given that there are multiple definitions of AI fairness that are mutually incompatible (Berk et al. 2018; Corbett-Davies et al. 2017; Kleinberg et al. 2017), fairness metrics must be decided upon within individual research domains; I argue for two fairness metrics relevant to the domain of sociolinguistic auto-coding. Second, I find empirical evidence for overlearning in sociolinguistic auto-coding by analyzing Villarreal et al.'s (2020) /r/ auto-coder. Third, I test a variety of unfairness-mitigation strategies on this data, finding substantial improvement with respect to fairness at the expense of predictive performance.
Bio: Dan Villarreal is a computational sociolinguist, as his scholarly work sits at the nexus of two research traditions: bringing together computational methods and sociolinguistic perspectives. In particular, his research seeks to expand sociolinguists' research toolkits by making computational techniques and sociolinguistic data accessible and usable; explore how speakers and listeners make sense of the tremendous phonetic variability that characterizes everyday speech; and foster a computational sociolinguistics (and a linguistics more broadly) that addresses its research questions faster, better, and more equitably. His recent work has investigated computational methods to automatically code sociophonetic variation (and how to make these methods equitable), gender segregation and speech communities in New Zealand, and whether Open Methods in linguistics contribute to academic colonialism. His research has been published in Language Variation and Change, Laboratory Phonology, and Language and Speech. Dan pronounces his last name /ˌvɪləɹiˈæl/.
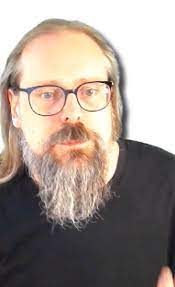
David Schlangen
University of Potsdam, Germany
Time: 12/10/2021, 12:30pm EST
Place: Zoom
Topic: Natural Language Processing Theory
Title: From Natural Language Processing to Natural Language Use
Abstract: This is a largely theoretical talk, in which I try to develop an argument for a particular research programme in "linguistic AI". The first step will be to identify the standard research programme in NLP, which is harder as it perhaps should be, as NLP (in its guise as engineering practice) doesn't tend to state or examine its presuppositions. I take "you can learn 'natural language understanding' from observation" and "you can atomise language use into seperately modelled ‘language tasks’ " to be two such presuppositions, and argue against them, in support for the claim that NLP, as it currently is set up, is limited to classification, transduction and compression (which natural language use goes beyond).
Using ideas from the philosophy of language on the role of norms in (linguistic) behaviour, I examine a number of cases where the straightforward application of NLP models in ways that make the resulting systems appear to be language users leads to problems, which can systematically be analysed as failures in normative behaviour. (Which to a certain degree can be addressed by adding explicit provisions to the system and/or its application context.) Highlighting one particular phenomenon, I argue that the speech act of assertion requires more than just being able to produce declarative sentences, even if they may seem situationally adequate; what is missing is a whole host of interactional capabilities.
This will bring me to an analysis of the prototypical interaction type, situated real-time interaction, as being built on what I call "the four cornerstones of linguistic intelligence": incremental processing, incremental learning, conversational grounding, and multimodal grounding; which separately and collectively form the targets of this research programme. As a further positive contribution, I argue for a focus on re-usable research objects (in addition to and beyond machine learning model architectures or "foundation models"), such as a) cognitive architectures, b) experiment environments, c) dialogue games. I close with a sketch of an evaluation framework for artificial language users: Collaborative Turing Games.
Many of the ideas in this talk are still somewhat speculative, so I look forward to your reactions.
Bio: David Schlangen is Professor of Computational Linguistics at the University of Potsdam, Germany. His main research interest is in language use and the interactive process of creating shared understanding through language use. He works both empirically on human/human dialogue, theoretically on formal modelling of the involved processes, and constructively on implementing language using agents in spoken dialogue systems and robots. He is the author of over 150 peer reviewed articles, with best paper awards at venues such as INLG and SIGdial.
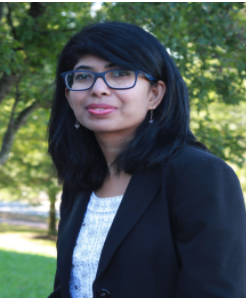
Samira Shaikh
University of North Carolina, Charlotte
Time: 11/12/2021, 12:30pm EST
Place: Zoom
Topic: Natural Language Generation
Title: On the evaluation of NLG systems
Abstract: Humans quite frequently interact with conversational agents. The rapid advancement in generative language modeling through neural networks has helped advance the creation of intelligent conversational agents. Researchers typically evaluate the output of their models through crowdsourced judgments, but there are no established best practices for conducting such studies. We look closely at the practices of evaluation of NLG output, and discuss implications of human cognitive biases on experiment design and the resulting data.
Bio: Samira Shaikh is an Assistant Professor in the Computer Science Department in the College of Computing and Informatics at the University of North Carolina - Charlotte (UNCC). She has a joint appointment with the Department of Psychology as an Assistant Professor in Cognitive Science, and is also an affiliate faculty member of the School of Data Science. She directs the SoLID Social Language and Intelligent Dialogue Agents Lab at UNCC. Her research interests are in the areas of Natural Language Processing, Data Science, Computational Sociolinguistics, Cognitive Science, and Artificial Intelligence, and she has extensive experience working in these areas of research through federally-funded grants as a Senior Research Scientist at the Research Foundation of SUNY. She received her PhD in Computer Science from the University at Albany - State University of New York in 2016. For her dissertation, she created a persuasive virtual chat agent, capable of changing people's opinion in online discussions. This research on persuasion, chatbots, and sociolinguistics has resulted in several active funded projects (DARPA, ARO, ONR and NSF) for which she currently serves as a PI and co-PI.
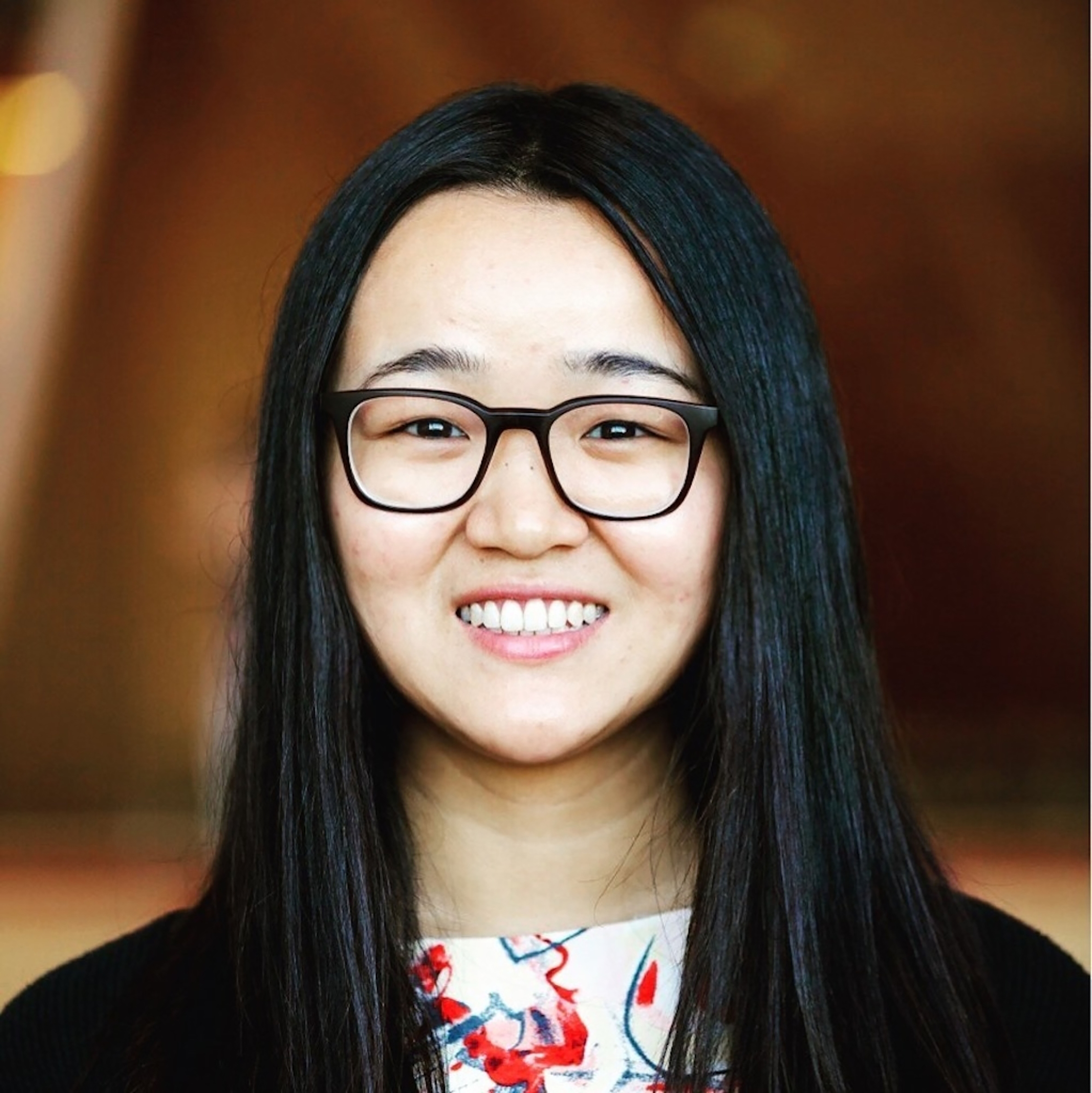
Diyi Yang
Georgia Institute of Technology
Time: 10/29/2021, 12:30pm EST
Place: Zoom
Topic: Socially-Aware NLP
Abstract: Natural language processing (NLP) has had increasing success and produced extensive industrial applications. Despite being sufficient to enable these applications, current NLP systems often ignore the social part of language, e.g., who says it, in what context, for what goals. In this talk, we take a closer look at social factors in language via a new theory taxonomy and its interplay with computational methods via two lines of work. The first one studies hate speech and racial bias by introducing a benchmark corpus on implicit hate speech and computational models on detecting and explaining latent hatred in language. The second part demonstrates how more structures of conversations can be utilized to generate better summaries for everyday interaction. We conclude by discussing several open-ended questions about how to build socially aware language technologies.
Bio: Diyi Yang is an assistant professor in the School of Interactive Computing at Georgia Tech, also affiliated with the Machine Learning Center (ML@GT), Institute for People and Technology (IPaT). She received her PhD from the School of Computer Science, Carnegie Mellon University. Diyi has broad interests in Natural Language Processing and Computational Social Science, including dialogue summarization, limited data learning, bias and hate speech, and NLP for social good. Her work has been published at leading NLP/HCI conferences and received multiple best paper awards (or nominations) at EMNLP, ICWSM, SIGCHI, and CSCW. She is a Microsoft Research Faculty Fellow, a Forbes 30 under 30 in Science, as well as a recipient of IEEE AI 10 to Watch and the Intel Rising Star Award.
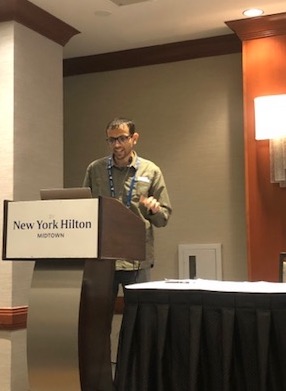
Varun Gangal
Carnegie Mellon University
Time: 10/15/2021, 12:30pm EST
Place: Zoom
Topic: Natural Language Generation
Bio: Varun is a PhD student at CMU LTI, advised by Eduard Hovy. His research is broadly on language generation, with specific interests in style transfer, data-to-text generation, narrative generation and low-resource & creative generation. He is also interested in Data Augmentation (DA), with specific interests in DA for generation, DA for better evaluating models and their robustness to domain shift. His work received the Best Long Paper award at INLG `21 and has appeared at ACL, EMNLP, NEURIPS and AAAI. He has co-organized the GEM workshop at ACL `21 and the upcoming CtrlGen workshop at NEURIPS `21.
Title1: Towards Endowing Generators with Style, Creativity and Commonsense
Abstract1: Over the span of this decade, NLG systems responding back, suggesting to and co-authoring language with humans have not just become technologically viable, but are now an accepted part of the social milieu in all geographies with basic levels of internet smartphone access. With generator models being deployed to a wider range of more specific applications, task specifications and user expectations increasingly require models to not just generate text which is content-adequate and fluent, but can also exhibit and be controlled to imbibe stylistic aspects and creative devices. In our work, we studied settings which required transferring or controlling for diachronic, persona and narrative-based style. Furthermore, we also devise NLG models for generating creative devices such as portmanteaus and figurative language. The newly improved fluency and adequacy of NLG model outputs has also brought to the fore previously occluded issues, a prominent one being poor commonsense plausibility in generated outputs, as shown by the CommonGen task (Lin et al 2020). Our work discovers two distinct mechanisms to improve the performance of SOTA generators such as BART and T5 on CommonGen. First, we show how models can "self-introspect" on initial outputs to expand input information, leading to more fluent and plausible final outputs. Second, we investigate the use of multimodal information contained in images as an effective method for enhancing the performance of generator models for Commongen. Our approach involves captioning images representing appropriate everyday scenarios, and using these captions to enrich and steer the generation process.
Title2: Data Augmentation for Finetuning and Evaluating Generators Better
Abstract2: First, we propose and evaluate GenAug, a suite of multiple augmentation methods, including some that incorporate external knowledge, for finetuning conditional generators in low-resource settings. Our experiments demonstrate that insertion of character-level synthetic noise and keyword replacement with hypernyms are effective augmentation methods, and outperform the randomized methods known to work well for text classification across several diversity and fluency metrics. Second, we investigate a seldom-explored use of data augmentation - to expand the set of references used to evaluate generators at test-time. We propose SCARCE, a novel technique for automatically expanding reference sets. We fetch plausible references from knowledge sources, and adapt them so that they are more fluent for the context in question. More specifically, we use (1) a commonsense KB to elicit a large number of plausible reactions given the context (2) relevant instances retrieved from corpus, using similar contexts. We demonstrate that our automatically expanded reference sets lead to large improvements in correlations of automated metrics with human ratings of system outputs for DailyDialog dataset.
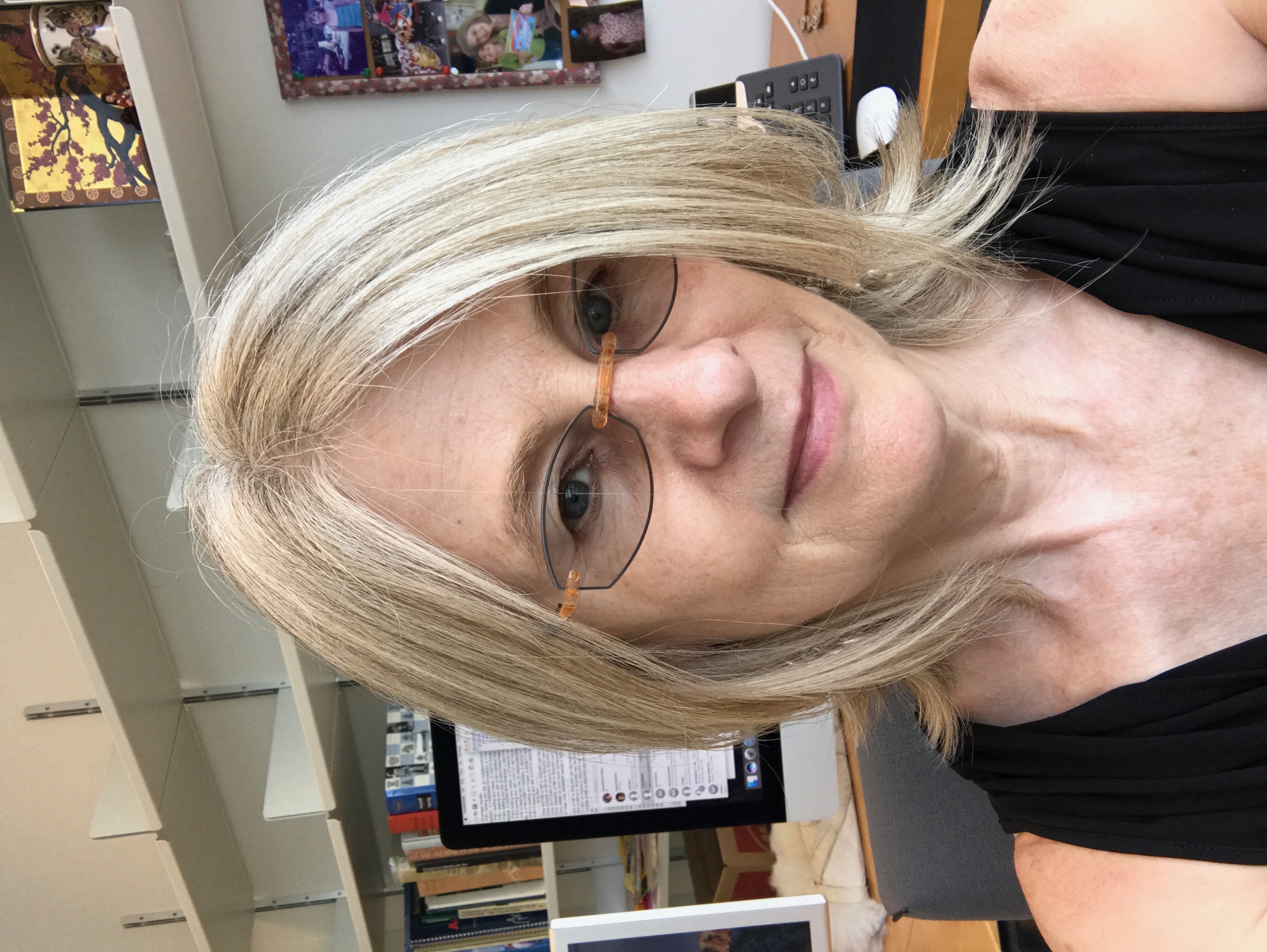
Rebecca Passonneau
Pennsylvania State University
Time: 10/8/2021, 12:30pm EST
Place: Zoom
Title: The ABCDs of NLP for Assessment of Student Writing
Topic: Educational Applications of NLP
Abstract: Application of natural language processing techniques to the assessment of student writing has the potential to alter current instructional methods for the better, to improve our understanding of the challenges for manual assessment, and not least, to provide new and interesting problems for NLP. The potential opportunity for NLP is that for at least a decade, educators have voiced concerns that students do not write well, that instructors’ primary training in subject matter education leaves them ill-equipped to help students write better, and that nevertheless, assessment of student writing remains an important vehicle for instructors to assess students’ understanding of ideas. Neither people nor machines can alone provide everything we want from writing assessment, but together they are likely to get closer to the ideal. The main hurdle to leap before NLP can achieve its potential in this arena is to provide interpretable assessment. In this talk, I discuss work from my lab that addresses interpretable assessment of student writing in the face of NLP’s reliance on uninterpretable models and representations.
Bio: Becky Passonneau has been a Professor in the Department of Computer Science and Engineering at Penn State University since 2016. Her research in natural language processing is on computational pragmatics. That is, she investigates how the same combinations of words have different meanings and intents in different contexts, and conversely, how the same meaning or intent can be expressed in different ways in a given context. She received her PhD in Linguistics from the University of Chicago in 1985, and worked at several academic and industry research labs before joining Penn State. Her work is reported in over 130 publications in journals and refereed conference proceedings, and has been funded through 27 sponsored projects from 14 sources, including government agencies, corporate sponsors, corporate gifts, and foundations.

Parisa Kordjamshidi
Michigan State University
Time: 9/24/2021, 12:30pm EST
Place: Zoom
Title: Exploiting Domain Knowledge and Relational Semantic Structures in Deep Learning for Natural Language Understanding
Topic: Domain Knowledge and Relational Semantics
Abstract: The recent research results in Natural Language Processing and many other complex problems show that deep learning models, for example transformer-based language models, trained on merely large volumes of data suffer from lack of interpretability and generalizability. While they might surprise us with writing an article that reads fluently given a few keywords, they can easily disappoint us by failing in some basic reasoning skills like understanding that “left” is the opposite direction of “right”. For solving real-world problems, we often need computational models that involve multiple interdependent learners, along with significant levels of composition and reasoning based on additional knowledge beyond available data. In my talk, firstly, I will discuss our recent research on developing deep learning techniques and architectures for solving NLP problems that 1) operate on structured semantic representations of data, 2) capture high order patterns in the data the enable relational reasoning, 3) consider domain knowledge in learning. Secondly, I will introduce our new Declarative learning-based programming framework, DomiKnowS, that is designed to help in the integration of learning and reasoning and exploiting both symbolic and sub-symbolic representations for solving complex and AI-complete problems. With this framework domain knowledge represented symbolically in the form of constraints can be seamlessly integrated in deep models using various underlying algorithms.
Bio: Parisa Kordjamshidi is an assistant professor of Computer Science & Engineering at Michigan State University. Her research interests are machine learning, natural language processing, and declarative learning-based programming. She has worked on the extraction of formal semantics and structured representations from natural language. She is an NSF CAREER award winner at 2019. She is the leading PI of a project supported by Office of Naval research to perform basic research and develop a declarative learning-based programming framework for integration of domain knowledge into statistical/neural learning. She obtained her Ph.D. from KU Leuven, in 2013 and was a post-doc in University of Illinois at Urbana-Champaign in cognitive computation group until 2016. She was a faculty member at Tulane University and a research scientist at Florida institute for Human and Machine Cognition between 2016-2019 before joining MSU. Kordjamshidi is a member of Editorial board of Journal of Artificial Intelligence Research (JAIR), a member of Editorial Board of Machine Learning and Artificial Intelligence, part of the journal of Frontiers in Artificial Intelligence and Frontiers in Big Data. She has published papers, organized international workshops and served as a (senior) program committee member or area chair of conferences such as IJCAI, AAAI, ACL, EMNLP, COLING, ECAI and a member of organizing committee of EMNLP-2021, ECML-PKDD-2019 and NAACL-2018 conferences.
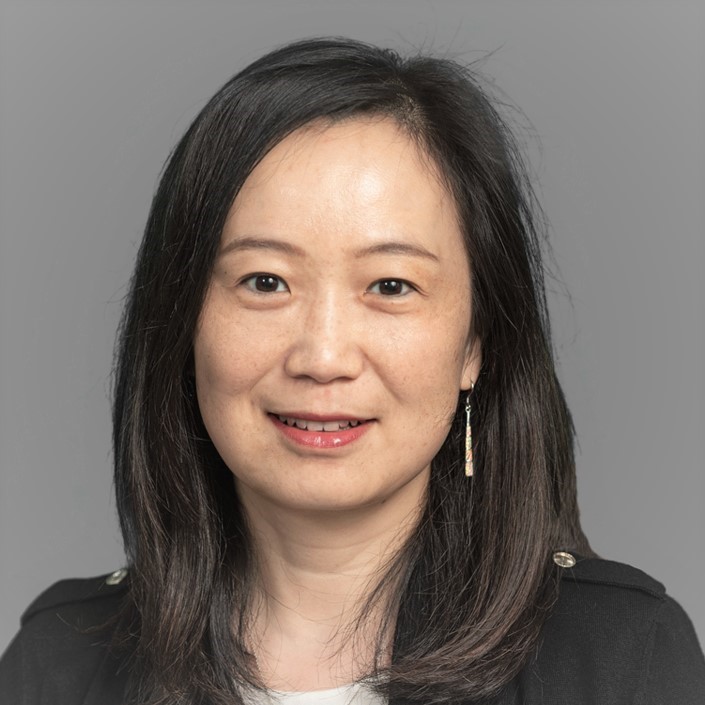
Joyce Chai
University of Michigan
Time: 4/20/2021, 1pm EST
Place: Zoom
Title: Language Communication with Robots
Topic: Human-Robot Communication
Abstract: With the emergence of a new generation of cognitive robots, enabling natural communication between humans and robots has become increasingly important. Humans and robots are co-present in a shared physical environment; however, they have mismatched capabilities in perception, action, and reasoning. They also have different levels of linguistic, world, and task knowledge. All of these lead to a significant disparity in their representations of the shared world, which makes language communication difficult. In this talk, I will introduce some effort in my lab that addresses these challenges, particularly in the context of communicative learning where humans can teach agents the shared environment and tasks through language communication. I will talk about collaborative models for grounded language processing which are motivated by cooperative principles in human communication. I will highlight the role of physical causality in grounding language to perception and action and discuss key challenges and opportunities.
Bio: Joyce Chai is a Professor in the Department of Electrical Engineering and Computer Science at the University of Michigan. Prior to joining UM in 2019, she was a Professor in Computer Science and Engineering at Michigan State University. Her research interests include natural language processing, situated and embodied dialogue, and human-AI communication and collaboration. Her recent work focuses on grounded language processing to facilitate natural communication with robots and other artificial agents. She is Associate Editor for Journal of Artificial Intelligence Research (JAIR) and ACM Transaction and Intelligent Interactive Systems (TiiS), and most recently served as Program Co-chair for the Annual Meeting of Association for Computational Linguistics (ACL) in 2020. She is the receipt of the National Science Foundation CAREER Award (2004), the Best Long Paper Award from Association of Computational Linguistics (2010), and the William Beal Outstanding Faculty Award at MSU (2018). She holds a Ph.D. in Computer Science from Duke University.

David Bamman
University of California, Berkeley
Time: 10/13/2020, Tuesday from 3 to 4 pm
Place: Zoom
Title: Modeling the Spread of Information within Novels
Topic: Language Generation
Abstract: Understanding the ways in which information flows through social networks is important for questions of influence--including tracking the spread of cultural trends and disinformation and measuring shifts in public opinion. Much work in this space has focused on networks where nodes, edges and information are all directly observed (such as Twitter accounts with explicit friend/follower edges and retweets as instances of propagation); in this talk, I will focus on the comparatively overlooked case of information propagation in *implicit* networks--where we seek to discover single instances of a message passing from person A to person B to person C, only given a depiction of their activity in text.
Literature in many ways presents an ideal domain for modeling information propagation described in text, since it depicts a largely closed universe in which characters interact and speak to each other. At the same time, it poses several wholly distinct challenges--in particular, both the length of literary texts and the subtleties involved in extracting information from fictional works pose difficulties for NLP systems optimized for other domains. In this talk, I will describe our work in measuring information propagation in these implicit networks, and detail an NLP pipeline for discovering it, focusing in detail on new datasets we have created for tagging characters and their coreference in text. This is joint work with Matt Sims, Olivia Lewke, Anya Mansoor, Sejal Popat and Sheng Shen.
Bio: David Bamman is an assistant professor in the School of Information at UC Berkeley, where he works in the areas of natural language processing and cultural analytics, applying NLP and machine learning to empirical questions in the humanities and social sciences. His research focuses on improving the performance of NLP for underserved domains like literature (including LitBank and BookNLP) and exploring the affordances of empirical methods for the study of literature and culture. Before Berkeley, he received his PhD in the School of Computer Science at Carnegie Mellon University and was a senior researcher at the Perseus Project of Tufts University. Bamman's work is supported by the National Endowment for the Humanities, National Science Foundation, an Amazon Research Award, and an NSF CAREER award.
Slides: Download
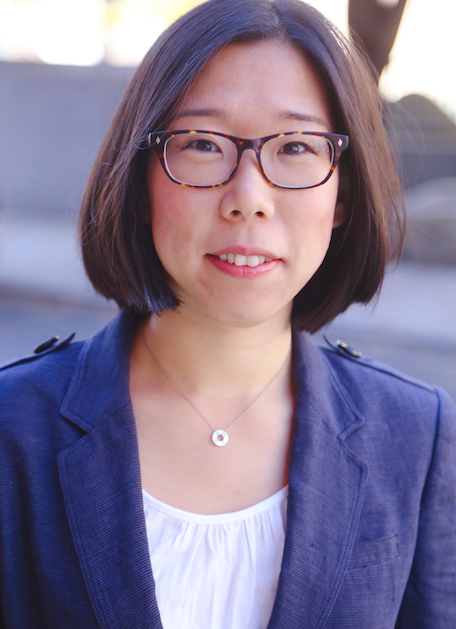
Wei Xu
Georgia Institute of Technology
Time: 10/27/2020, Tuesday from 3 to 4 pm
Place: Zoom
Title: Automatic Text Simplification for K-12 Students
Topic: Language Generation
Abstract: Reading and writing are fundamental to the learning experience of students. In this talk, I will first exemplify how professional editors rewrite news articles to meet readability standards of elementary and middle schools, then demonstrate how we can develop new machine learning models to mimic the human editing process.
I aim to answer four research questions: (1) How to create a parallel corpus for training neural text generation models? (2) How to design neural generation models with better controllability? (3) How to automatically evaluate system generated text outputs? (4) How to estimate the readability at the word- and phrase-level more reliably?
On the high-level, we designed a neural Conditional Random Fields model to automatically align sentences between the complex and simplified articles, and consequently, created two large text simplification corpora (Newsela-Auto and Wiki-Auto). We also proposed a novel hybrid approach that leverages linguistically motivated rules for splitting and deletion, and couples with a neural paraphrasing model to produce varied rewriting styles. SARI, a tunable automatic evaluation metric, has been used for system comparison in addition to human evaluation. As for readability assessment, we improved the state-of-the-art by using a pairwise neural ranking model in conjunction with a manually rated word-complexity lexicon.
Bio: Wei Xu is an assistant professor in the School of Interactive Computing at the Georgia Institute of Technology. Before joining Georgia Tech, she was an assistant professor at Ohio State University since 2016. Xu’s research interests are in natural language processing, machine learning, and social media. Her recent work focuses on text generation, semantics, information extraction, and reading assistive technology. She has received the NSF CRII Award, Best Paper Award at COLING, CrowdFlower AI for Everyone Award, and Criteo Faculty Research Award.
Slides: Download
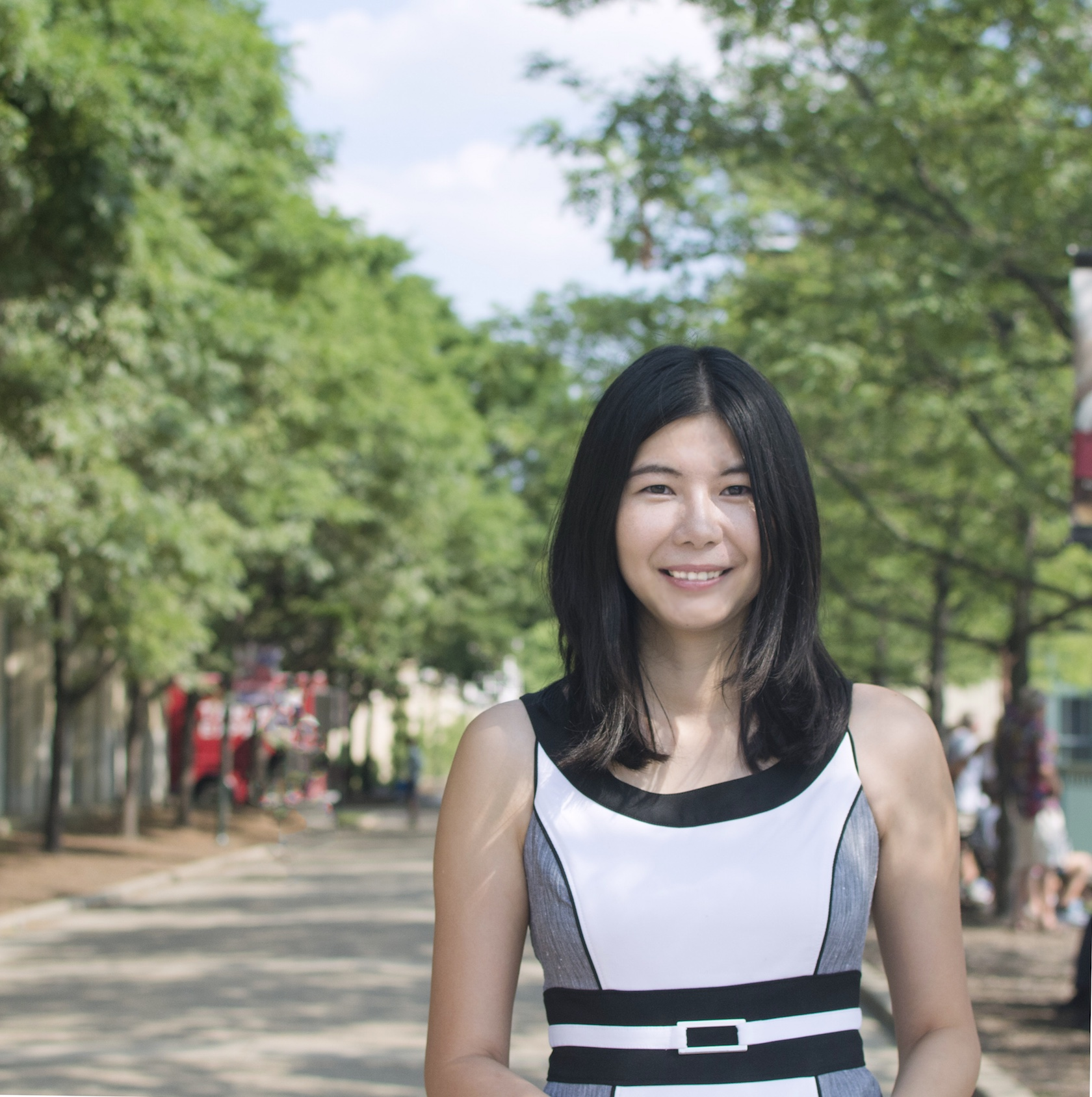
Zhou Yu
University of California, Davis
Time: 11/10/2020, Tuesday from 3 to 4 pm
Place: Zoom
Title: Personalized Persuasive Dialog Systems
Topic: Dialogue Systems
Abstract: Dialog systems such as Alexa and Siri are everywhere in our lives. They can complete tasks such as booking flights, making restaurant reservations and training people for interviews. These systems are passively follow-along human needs. What if the dialog systems have a different goal than users. We introduce dialog systems that can persuade users to donate to charities. We further improve the dialog model's coherence by tracking both semantic actions and conversational strategies from dialog history using finite-state transducers. Finally, we analyze some ethical concerns and human factors in deploying personalized persuasive dialog systems.
Bio: Zhou Yu is an Assistant Professor at the UC Davis Computer Science Department. Zhou will join the CS department at Columbia University in Jan 2021 as an Assistant Professor. She obtained her Ph.D. from Carnegie Mellon University in 2017. Zhou has built various dialog systems that have a real impact, such as a job interview training system, a depression screening system, and a second language learning system. Her research interest includes dialog systems, language understanding and generation, vision and language, human-computer interaction, and social robots. Zhou received an ACL 2019 best paper nomination, featured in Forbes 2018 30 under 30 in Science, and won the 2018 Amazon Alexa Prize.
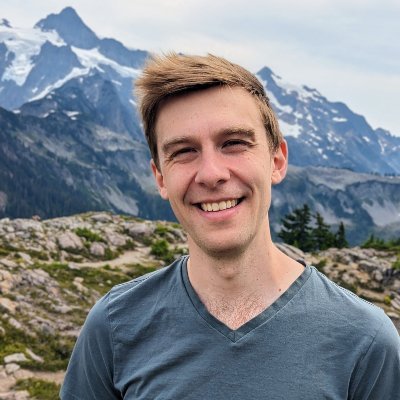
Jack Hessel
AI2
Time: 11/24/2020
Place: Zoom
Title: (at least) Two Conceptions of Visual-Textual Grounding
Topic: Multimodal Communication
Abstract: Algorithms that learn connections between visual and textual content underlie many important applications of AI, e.g., image captioning, robot navigation, and web video parsing. But what does it really mean for images and text to be "connected"? I'll discuss (at least) two orthogonal conceptions of visual-textual grounding. The first is operational grounding: if an algorithm can learn a consistent relationship between two data modalities based on co-occurrence data, then such a pattern can be called "grounded;" I'll discuss our work that applies this notion to both static images and to web videos. The second, more general view describes visual-textual grounding as a subset of "interesting" logical functions that take as input visual and textual variables. Under this paradigm, we design a diagnostic that can tell you if your multimodal model is doing cross-modal reasoning, or (as we find is the common case) exploiting single modal biases.
Bio: Jack is a postdoc at AI2, and earned a PhD in Computer Science at Cornell University. His work focuses on analyzing user-generated web content, and has been published at EMNLP, NAACL, WWW, etc. Previously, he's worked at Google, Facebook, and Twitter, and held an invited visiting faculty position in Computer Science at Carleton College.
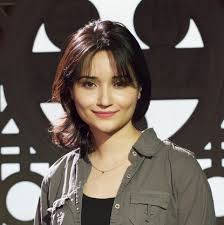
Nasrin Mostafazadeh
Verneek
Time: 2/9/2021
Place: Zoom
Title: How far have we come in giving our NLU systems common sense?
Topic: Common Sense Reasoning
Abstract: Commonsense reasoning has been a long-established area in AI for more than three decades. Despite the lack of much ongoing effort in this area after the 80s, recently there has been a renewed interest in the AI community for giving machines common sense, acknowledging it as the holy grail of AI. With the tremendous recent progress in natural language understanding (NLU), the lack of commonsense reasoning capabilities of NLU systems is more evident than ever. In this talk, I’ll discuss the amazing recent progress made in tackling commonsense reasoning benchmarks using the gigantic pre-trained neural models. I’ll talk about the role of benchmarks in measuring our progress and how we can move the goal post. Constructing coherent mental models of narratives that an NLU system reads, through establishing the chain of causality of implicit and explicit events and states, is a promising step forward.
Bio: Nasrin is Co-founder of Verneek, a new AI startup that is striving to enable anyone to make data-informed decisions without needing to have any technical background. Before Verneek, Nasrin held senior research positions at AI startups BenevolentAI and Elemental Cognition and earlier at Microsoft Research and Google. She received her PhD at the University of Rochester working at the conversational interaction and dialogue research group under James F. Allen, with her PhD work focused on commonsense reasoning through the lens of story understanding. She has started lines of research that push AI toward deeper understanding and having common sense, with applications ranging from storytelling to vision & language. She has been a keynote speaker, chair, organizer, and program committee member at different AI venues. Nasrin was named to Forbes’ 30 Under 30 in Science 2019 for her work in AI.
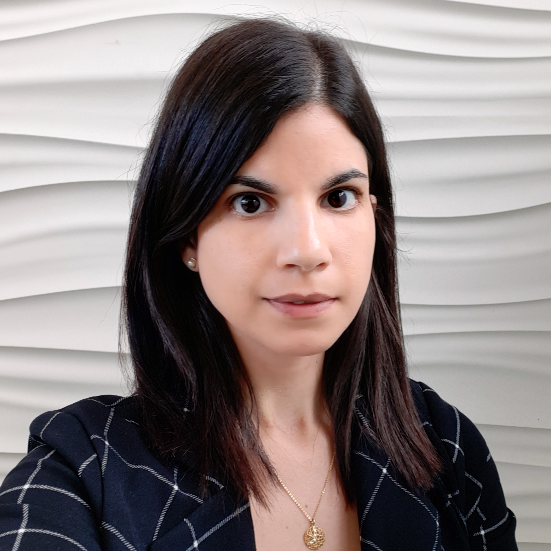
Vered Shwartz
AI2
Time: 2/23/2021
Place: Zoom
Title: Commonsense Knowledge and Reasoning in Natural Language
Topic: Common Sense Reasoning
Abstract: Natural language understanding models are trained on a sample of the real-world situations they may encounter. Commonsense and world knowledge, language, and reasoning skills can help them address unknown situations sensibly. In this talk I will present two lines of work addressing commonsense knowledge and reasoning in natural language. I will first present a method for discovering relevant knowledge which is unstated but may be required for solving a particular problem, e.g., to correctly resolve "Children need to eat more vegetables because they [children / vegetables] are healthy" one needs to know that "vegetables are healthy". Such knowledge is discovered through a process of asking information seeking clarification questions (e.g. "what is the purpose of vegetables?") and answering them ("to provide nutrients"). I will then discuss nonmonotonic reasoning in natural language, a core human reasoning ability that has been studied in classical AI but mostly overlooked in modern NLP. I will talk about several recent papers addressing abductive reasoning (reasoning about plausible explanations), counterfactual reasoning (what if?) and defeasible reasoning (updating beliefs given additional information). Finally, I will discuss open problems in language, knowledge, and reasoning.
Bio: Vered Shwartz is a postdoctoral researcher at the Allen Institute for AI (AI2) and the Paul G. Allen School of Computer Science & Engineering at the University of Washington. Previously, she completed her PhD in Computer Science from Bar-Ilan University, under the supervision of Prof. Ido Dagan. Her research interests include commonsense reasoning, lexical and compositional semantics.
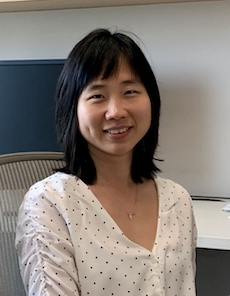
Jessy Li
UT Austin
Time: 3/23/2021
Place: Zoom
Title: Help! Need Advice on Discourse Comprehension
Topic: Discourse Comprehension
Abstract: With large-scale pre-trained models, natural language processing as a field has made giant leaps in a wide range of tasks. But how are we doing on those that require a deeper understanding of discourse pragmatics, tasks that we humans use language to accomplish on a daily basis? We discuss a case study of advice giving in online forums, and reveal rich discourse strategies in the language of advice. Understanding advice would equip systems with a better grasp of language pragmatics, yet we show that advice identification is challenging for modern NLP models. So then --- how do people comprehend at the discourse level? We tackle this via a novel question generation paradigm, by capturing questions elicited from readers as they read through a text sentence by sentence. Because these questions are generated while the readers are processing the information, they are naturally inquisitive, with a variety of types such as causal, elaboration, and background. Finally, we briefly showcase a new task that requires high level inferences when the target audience of a document changes: providing elaborations and explanations during text simplification.
Bio: Jessy Li (https://jessyli.com) is an assistant professor in the Department of Linguistics at UT Austin where she works on computational linguistics and natural language processing. Her work focuses on discourse organization, text intelligibility, and language pragmatics in social media. She received her Ph.D. in 2017 from the University of Pennsylvania. She received an ACM SIGSOFT Distinguished Paper Award at FSE 2019, an Area Chair Favorite at COLING 2018, and a Best Paper nomination at SIGDIAL 2016.
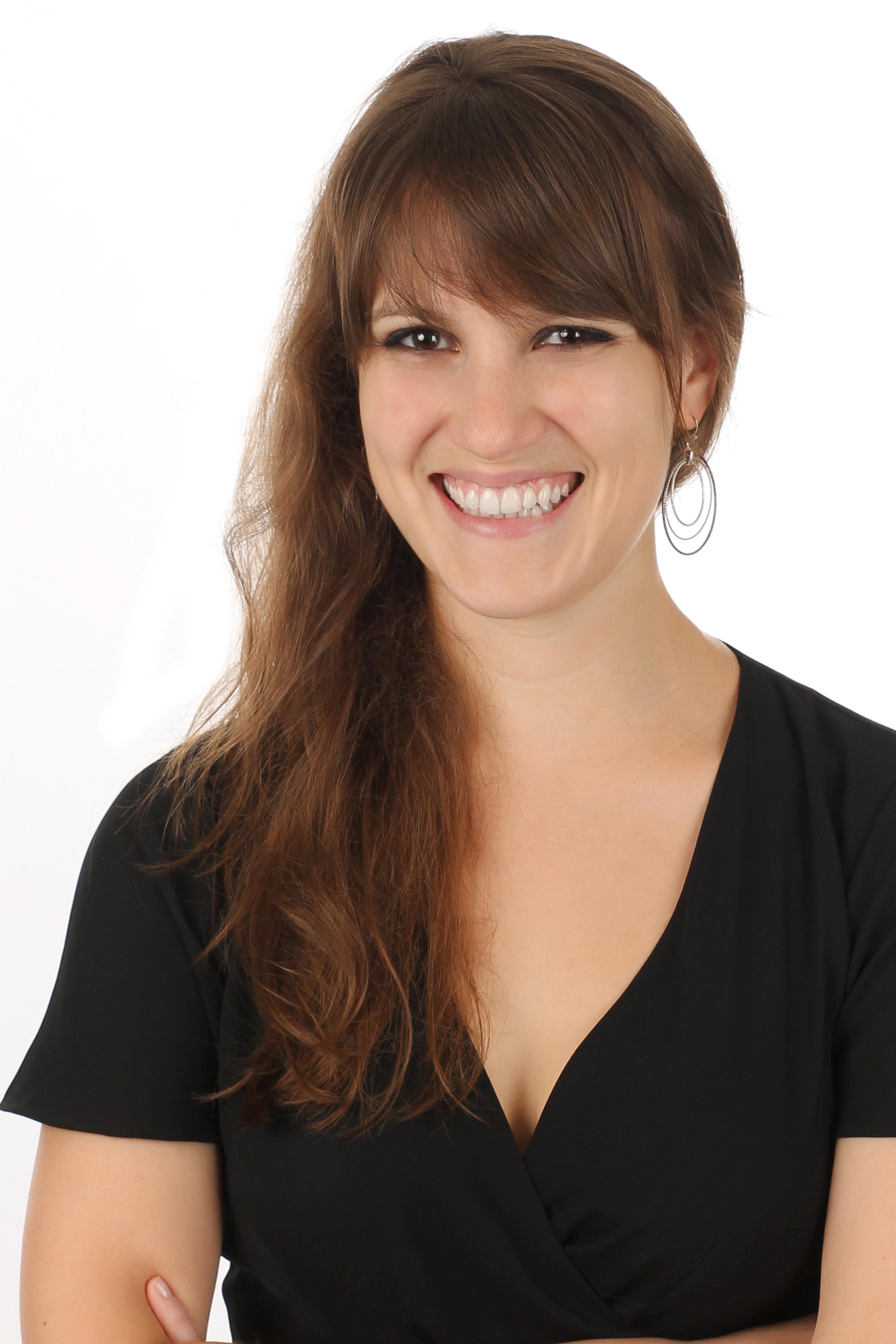
Ellie Pavlick
Brown University
Time: 4/6/2021
Place: Zoom
Title: You can lead a horse to water...: Representing vs. Using Features in Neural NLP
Topic: Pretrained Language Models
Abstract: A wave of recent work has sought to understand how pretrained language models work. Such analyses have resulted in two seemingly contradictory sets of results. On one hand, work based on "probing classifiers" generally suggests that SOTA language models contain rich information about linguistic structure (e.g., parts of speech, syntax, semantic roles). On the other hand, work which measures performance on linguistic "challenge sets" shows that models consistently fail to use this information when making predictions. In this talk, I will present a series of results that attempt to bridge this gap. Our recent experiments suggest that the disconnect is not due to catastrophic forgetting nor is it (entirely) explained by insufficient training data. Rather, it is best explained in terms of how "accessible" features are to the model following pretraining, where "accessibility" can be quantified using an information-theoretic interpretation of probing classifiers.
Bio: Ellie Pavlick is an Assistant Professor of Computer Science at Brown University where she leads the Language Understanding and Representation (LUNAR) Lab. She received her PhD from the one-and-only University of Pennsylvania. Her current work focuses on building more cognitively-plausible models of natural language semantics, focusing on grounded language learning and on sample efficiency and generalization of neural language models.